I have been following OpenAI’s large language models since the summer of 2022, when GPT-3 was released. Even at that time, GPT-3 demonstrated occasional glimpses of its potential to assist in dispute resolution. For instance, it spontaneously performed an expected value calculation, which it used to help make a decision on whether to settle or proceed to trial.
Back then, you could input a couple of sentences and get a few sentences in return. As the context window and overall sophistication of models has grown, it is now possible to upload hundreds of pages of documents and get very long and thoughtful responses back. Google’s NotebookLM, for example, can read a 500-page mediation bundle and give you back a summary of the key points in the dispute and a timeline of key events.
But if you just ask the model to act as a judge or arbitrator or mediator, you will generally get a defensive reply along the lines of “I am a large language model. I am not a lawyer. You need to consult your own lawyer in order to obtain accurate advice about how to settle this dispute, blah, blah.”
I had tried this a few times and never really made much progress.
Then I came across this paper in Science: “AI can help humans find common ground in democratic deliberation”
By serendipitous coincidence, I have a distant connection with one of the authors, Professor Chris Summerfield, a professor of cognitive neuroscience at Oxford and a member of the UK AI Safety panel. I got in touch with him to discuss his paper, and he kindly made time to chat. Chris explained how his team approached their challenge: they focused on politically charged questions (e.g., “Should we have capital punishment?” or “Should we invest more in rail travel?”) where opinions often diverge. Their method involved having the AI generate a series of reports, each iteration bringing the opposing sides closer to consensus. This process continued until the highest level of agreement was achieved. The AI slightly outperformed human mediators in this task, though the margin was modest.
Could AI do something similar with commercial disputes? It turns out that it can. I have now assembled a series of prompts which gently walk an AI through a thought process taking it from providing an initial summary of a dispute, through to considering possible outcomes if it goes to trial, then estimating the probability of those outcomes, then working through an expected value calculation, before finally suggesting a settlement figure for the two sides to consider.
As I have worked with large language models over the past few years, there have been plenty of occasions when I have literally sat in open-mouthed astonishment at the screen. Seeing GPT3 spontaneously generate an expected value calculation back in July 2022 was one such moment.
This was another: out of twelve anonymised disputes that I used in my recent testing, the model (once I had finessed the prompts with sufficient care) proposed a settlement figure which was, in every case, within 10% of the figure that the two sides settled at.
This is extraordinary. There is no way the model could have known where the two sides actually settled. The parties have reached that figure after months of negotiation and hours of mediation. The AI is reaching it just from reading the bundle.
All I did in this experiment was focus on careful prompting, and on figuring out a series of intermediate steps which would help the AI feel its way to a conclusion. I didn’t perform any fine-tuning on the model. (Fine-tuning involves providing the model with additional training on material specific to the domain of interest—such as property law or negotiation strategies, for example.) With fine-tuning added, it would surely be possible to create a model capable of predicting where two sides would settle with even more astonishing accuracy.
In an article I wrote in 2022 about GPT-3 I made this prediction which has turned out to be wrong:
“In another five years’ time, it seems to me that we will be able to give GPT-3 (or a similar system) a huge bundle of evidence relating to Alice and Bob’s dispute and it could provide a useful summary of the issues and provide reliable advice on how to resolve the dispute. It will certainly be able to do a reliable expected value calculation to set parameters for settlement discussions. And I would expect it to be able to distil what the key points of agreement and disagreement are, to help focus negotiations on those points.”
I was wrong because only two years have passed, not five.
Is AI-powered dispute resolution actually useful, though? Maybe not yet: I don’t think the world is ready for it.
At the moment, most people are not using AI in their day to day lives and are mostly unaware of how sophisticated it has become. If the disputing parties are presented with what they think is a number which the AI has plucked from thin air, they are unlikely to take it seriously. Part of the power of a drawn-out negotiation is that you come to understand that the offer on the table late at night after a long day of mediation is likely to be the best offer you will see.
I am confident that the number which the AI is suggesting is also very likely to be the best offer you will see, but most people would not have faith in that, I suspect.
This dynamic is likely to shift as AI becomes more integrated into professional services over the next few years. As lawyers and mediators begin incorporating AI tools into their daily workflow – first for document review and case analysis, then gradually for more sophisticated tasks – clients will become more accustomed to seeing and trusting AI-generated insights. The tipping point may come when major law firms and ADR providers begin openly advertising their use of AI to enhance their services, much as they did with e-discovery tools a decade ago. At that stage, having an AI assessment of settlement parameters could become not just accepted, but expected as part of the standard dispute resolution toolkit.
There may be particular scenarios where this would work now, though. Situations which currently use “interim binding” adjudications might be one such area. In construction disputes, an adjudicator’s decision can be binding on an interim basis, meaning the parties agree to accept it and continue working as if the decision is binding, but it is possible to later have the decision overturned by a court, arbitrator, or by agreement. This is to allow construction to continue swiftly, but with the fallback that one side could eventually seek to overturn the decision if they wanted to.
It also might be possible to take the disputing parties through a process which gives them confidence in the AI, before they see the proposed settlement figure. The AI could give them a short summary report, followed by a more sophisticated analysis, and so on, to establish credibility before offering to propose a settlement figure. This is the approach I am taking with the experimental service I have called Claudius ADR.
Are there other areas where the world might be ready to allow AI to help them resolve their disputes?
________________________
To make sure you do not miss out on regular updates from the Kluwer Mediation Blog, please subscribe here.
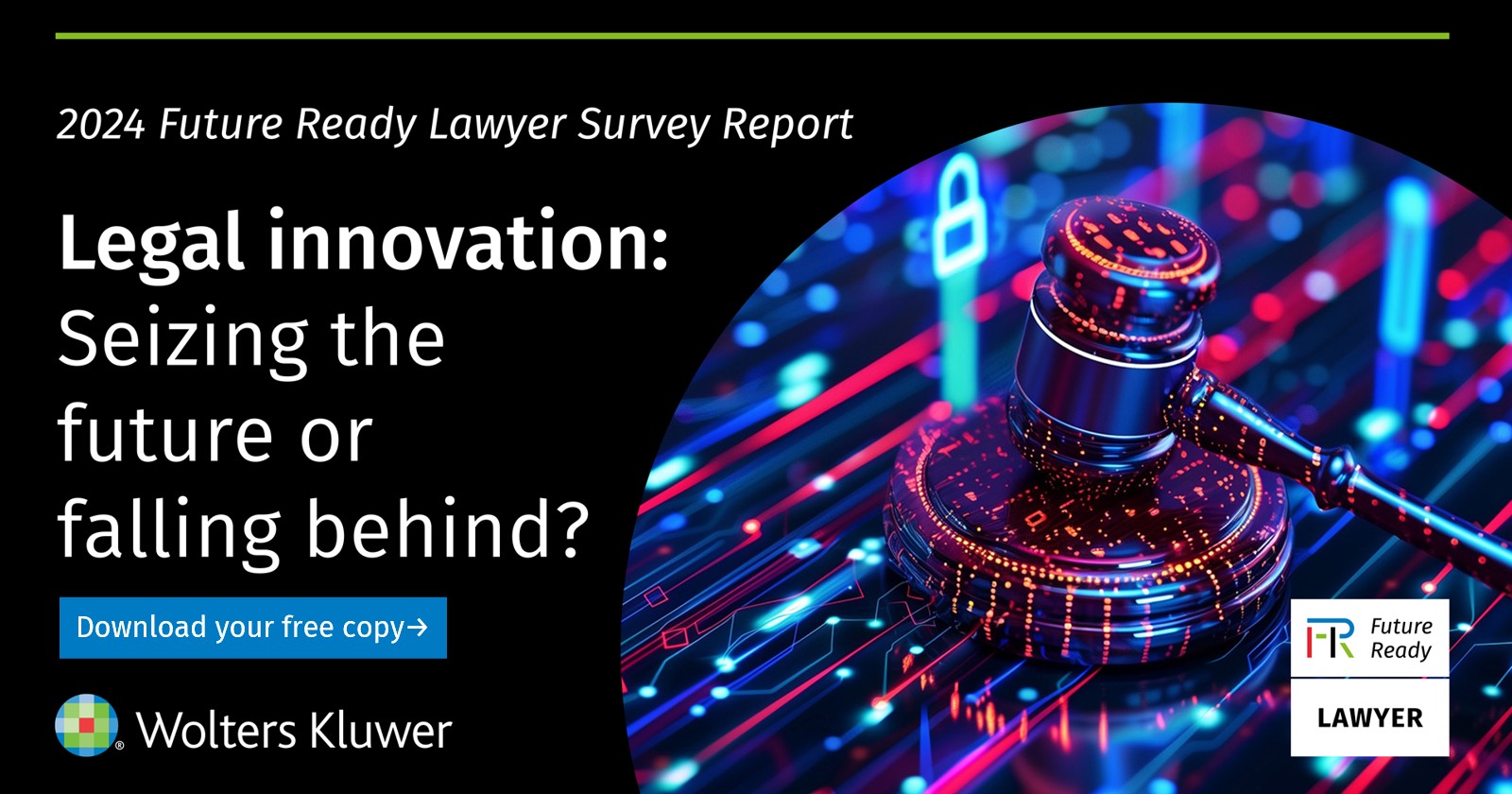

Editorial Comment: Is the Mediation Community Ready for AI?
This compelling and thought-provoking post captures the awe—and the caution—with which many of us are approaching the integration of AI into dispute resolution. The author’s ability to distil real-world experimentation with language models into an accessible narrative is striking. From GPT-3’s tentative beginnings to current models accurately predicting settlement figures within 10% of final outcomes, the progress is not just impressive—it is transformative.
What stands out is the subtle shift in role for AI: not as a judge, lawyer or even mediator, but as a highly analytical, neutral assistant—capable of summarising, pattern-finding, calculating expected outcomes, and proposing reasonable offers. As the author rightly notes, trust in the process—so central to mediation—may be the biggest hurdle. Yet, history suggests that once tools become embedded in professional practice (as e-discovery once did), acceptance follows rapidly.
This leads us to a question we’d love our readers to weigh in on:
Where do you see the most immediate or promising use cases for AI in mediation practice?
Is it in pre-mediation briefing and document analysis? As a co-pilot in joint session preparation? Or perhaps as an independent evaluator of BATNA/WATNA and settlement ranges?
We welcome your views and experiences—especially any experiments, small or large, that you’ve conducted using AI in your own mediation work. As AI continues to develop at speed, the mediation community’s collective insight will be crucial in shaping its responsible, ethical, and effective integration.