Most people’s experience of artificial intelligence (AI) is limited to interacting with a chat bot on their bank’s website. These bots are unimpressive, dispensing trivial and mostly unhelpful answers.
Don’t be misled into thinking that unhelpful chat bots represent the state of the art in AI, though. The cutting edge of AI research has moved far beyond what you see in everyday life.
Consider OpenAI’s GPT-3. GPT-3 works like a very powerful auto-complete system. Everyone is familiar with their phone offering to auto-complete each word as you type a text message. If you use Gmail, you will have seen Gmail’s ability to auto-complete entire sentences.
GPT-3 auto-completes entire documents, given a few words or sentences to start it off. It works by figuring out what the most likely next word is, and the word after that, and so on, until it has written a few paragraphs of text.
I recently signed up for an account and had a play around. I had seen some astonishing examples of what GPT-3 can produce circulating on social media, so I wanted to see for myself what it could do.
Here is what I provided as a prompt:
“Alice has a dispute with Bob. If Alice wins at trial, she will win £500,000. If she loses, she will have to pay £100,000 in legal fees. Alice’s lawyer says that she has a 65% chance of winning. Should Alice continue to trial, or should she try and settle?”
GPT-3’s initial response was rather underwhelming, saying simply that “Alice should continue to trial”:
(In all of these screenshots, the unhighlighted text is what I have typed. The green highlighted text is what GPT-3 generated in response to my typed prompt).
So I submitted the same prompt a second time. This time GPT-3 opened up and offered a bit more:
“There is no easy answer to this question. If Alice settles, she will most likely receive a smaller sum of money than if she wins at trial, but she will also avoid the risk of having to pay legal fees. If Alice goes to trial, she has a higher chance of winning a larger sum of money, but she also runs the risk of losing and having to pay legal fees. Ultimately, the decision of whether to settle or go to trial depends on Alice’s risk tolerance and her assessment of the chances of winning.”
Wow. This wouldn’t win over a TED talk audience, but it is still quite remarkable for a robot to generate this text. Each sentence here is a novel sentence – this isn’t just a matter of GPT-3 stumbling across some relevant text on the internet and regurgitating it back again. It gives the impression of really understanding the prompt and providing a considered answer. As we will see later, though, GPT-3 doesn’t really understand anything at all, although it sometimes gives a very convincing impression of someone who does understand.
I ran it a few more times and got some different answers in a similar vein. Then this answer made me splutter on my tea:
“If Alice’s expected value from going to trial is positive, she should continue to trial. If her expected value is negative, she should try and settle.
Expected value = (0.65)(500,000) – (0.35)(100,000) = 325,000 – 35,000 = 290,000
Since Alice’s expected value is positive, she should continue to trial”
This is mind-blowing. I didn’t tell GPT-3 to do an expected value calculation. Yet it does this spontaneously, and correctly, and shows its workings like a good student.
It is interesting that the final sentence is not correct, however. Alice should compare the expected value of going to trial with the expected value of settling. It is as if GPT-3 is bluffing, and hoping that what it says is correct, but it doesn’t really know. Indeed, this is exactly what it is doing.
That aside, this response seems incredibly sophisticated to me. To think that the same basic principles which underpin your phone’s auto-complete function can generate a response like this, is stunning to me.
However, sometimes GPT-3 says things which are not only wrong, but really nonsensical, like this answer:
“Alice should try and settle because she has a 65% chance of winning, which means she has a 35% chance of losing. Losing at trial would mean having to pay £100,000 in legal fees which is more than the £500,000 she would win if she won the trial.”
Again, this sounds fluent and convincing at first glance, but it doesn’t quite make sense. £100,000 is definitely not “more than” £500,000.
And this one:
“The expected value of going to trial is £325,000, which is greater than the expected value of settling, which is £200,000. Therefore, Alice should continue to trial.”
Where does the £200,000 figure come from? No idea.
I also asked it to talk about mediation case law. I gave it this prompt, taken from a genuine, human-authored summary of Halsey, a well-known Court of Appeal case relating to a party refusing to mediate:
“In Halsey v Milton Keynes General NHS Trust (Halsey), the Court of Appeal established that an unsuccessful litigant has the burden of demonstrating why the court should depart from the general rule set out in CPR 44.2. It considered the following non-exhaustive factors to be relevant to the court’s assessment of the parties’ conduct and, in particular, whether a party has unreasonably refused to engage in ADR (having regard to all the circumstances of the case):”
GPT-3 finished this summary by generating over 800 words of extremely convincing, but entirely false, narrative about Halsey and its implications. It is too long to reproduce here, and I fear that by placing the actual text here some careless law student will stumble across it and think it is an accurate summary.
So here is an image of some of the response:
(I have put the full text generated by GPT-3 on my blog here, with a suitable health warning, if you want to read it in full.)
Note how the response begins with a list of key points, and the main text is divided into sections, each with a heading which matches one of those key points. It is a well-structured, well-written document.
Note also the total fiction employed in describing the claimant and defendants:
“The defendants in Halsey were four NHS Trusts. The claimant, a consultant obstetrician, had separate claims against each of them for breach of contract, sexual discrimination and victimisation.”
The claimant in Halsey was the family of a deceased patient. There was only one defendant, the Milton Keynes trust. The description of the case also bears no relation whatsoever to the actual facts of the Halsey case. It is almost entirely a work of fiction.
This pattern persists. I asked it to summarise another well-known case involving mediation, Wright vs Michael Wright Supplies, and it again generated a very convincing, but entirely fictional, response.
The response is frighteningly convincing, but quite wrong. Wright has nothing to do with ACAS, and it is not even an employment dispute.
What should we make of this?
Ten years ago, it would have seemed to me that the hardest AI challenge would be to teach a computer to write fluent, well-structured text. When to use bullet points, when to use paragraph headings, how to structure a piece of writing so that it has an introduction, a middle and an end. You would think that would be much harder than teaching the computer some facts.
But it seems that the ‘writing fluently’ challenge has already been solved. Over and over again, GPT-3 can produce well-written answers. It can even write in a particular style. Here, I asked it to write about “access to justice” in the style of a populist leader, and then in the style of an academic:
I would say that the writing problem is solved. As we have seen, GPT-3 is quite good at writing fiction.
In contrast, our everyday experiences tell us that computers are quite good at giving you facts. If you ask Alexa or Siri a simple fact-based question (“what was the score in today’s City v Villa match?”) you will get an accurate answer.
It feels to me that we are really very close indeed to having a system which can both write fluently and accurately. In another five years’ time, it seems to me that we will be able to give GPT-3 (or a similar system) a huge bundle of evidence relating to Alice and Bob’s dispute and it could provide a useful summary of the issues and provide reliable advice on how to resolve the dispute. It will certainly be able to do a reliable expected value calculation to set parameters for settlement discussions. And I would expect it to be able to distil what the key points of agreement and disagreement are, to help focus negotiations on those points.
And it doesn’t seem, to me, to be such a long way to actually asking a sufficiently advanced robot to rule on a dispute. Perhaps we would first start to rely on robots to make “interim binding” decisions. Interim binding decisions are used in construction arbitrations in the UK: the parties agree to be bound by the (human) arbitrator’s decision unless or until one party goes to court and has the decision overturned. In practice, the parties mostly just accept the decision and do not challenge it in court. Using that same mechanism would allow us to test the water with robot arbitrators, perhaps.
We don’t yet know what the next generation of GPT-3 will be able to do. But let me leave you with what GPT-3 thinks the future holds, and maybe this time it is not writing fiction:
____________
Useful links:
Halsey: https://www.bailii.org/cgi-bin/format.cgi?doc=/ew/cases/EWCA/Civ/2004/576.html
Wright: https://www.bailii.org/cgi-bin/format.cgi?doc=/ew/cases/EWCA/Civ/2013/234.html
GPT-3: https://openai.com/api/
Connect with me on LinkedIn: http://www.linkedin.com/in/brucegreig
________________________
To make sure you do not miss out on regular updates from the Kluwer Mediation Blog, please subscribe here.
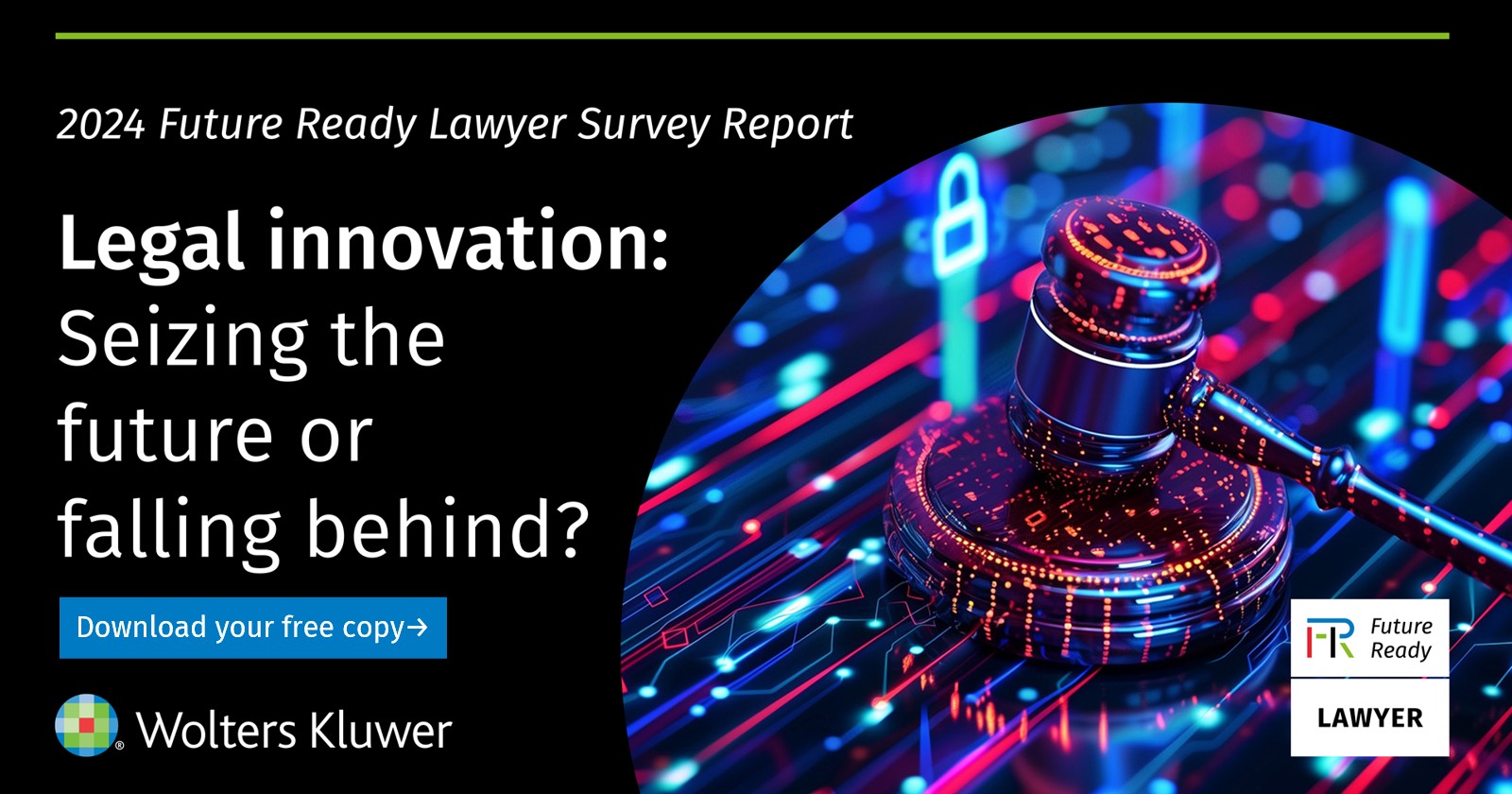

A very good article, thank you